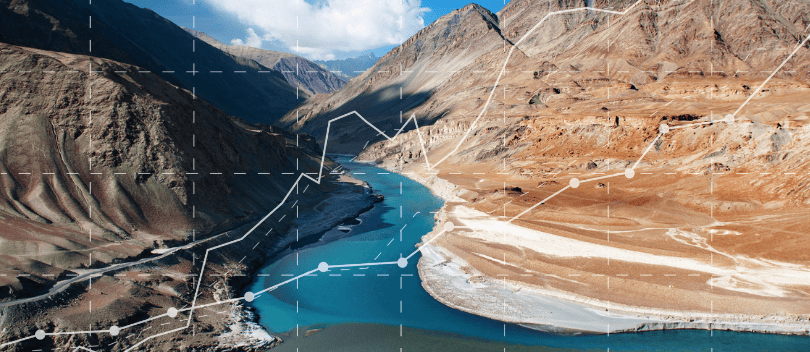
Best Practice Approach to Stage-Discharge Rating Curve Development
The rate of downloads of the paper “5 Best Practices for Building Better Stage-Discharge Rating Curves” indicates a very high latent demand for guidance on how to develop rating curves.
A stage-discharge rating curve represents the relation of water level at a given point in a stream to a corresponding volumetric rate of flow.
The shape of a curve can be discovered by conducting synchronized measurements of stage and discharge and investigating the pattern of points on a scatter plot. Any physical change to the stream channel will alter this relation and these changes must be accounted for in the derivation of a discharge hydrograph from a time series of stage data.
In an ‘ideal’ world there would always be enough measurements to empirically characterize the shape of the curve for every channel configuration. In the ‘real’ world obtaining ‘enough’ measurements would require an enormous investment in stream gauging.
There are often only enough measurements to hint at what the true shape of the curve is and how it changes over time. The real skill in rating curve development is in interpreting these clues to disclose the truth.
The street work method of detective work requires a senior hydrographer with powers of deduction informed by many years of experience. The forensic method of detective work is an evidence based approach that uses the scientific method to deduce the truth from sparse evidence.
Both methods are valid but there are fewer and fewer hydrographers with the Columbo-like eye for detail and intuition to ask ‘just one more question’ needed to get at the underlying truth. Use of the scientific method can help hydrometric agencies cope with a changing demographic.
A poorly-conceived rating curve can produce discharge data that does not pass the test of hydrologic reason.
Extreme values from curve extrapolation may be too high (e.g. a rainfall runoff ratio > 1), or too low. The early years of record may have peaks defined by a badly extrapolated curve relative to ‘better’, more mature, extrapolations resulting in an apparent (but not real) trend in peak flows. The seasonal water balance may be skewed by poorly modeled backwater effects.
Developers of hydrologic models are particularly attuned to whether the discharge time series make hydrologic sense or not.
Calibration of model parameters to force a fit to unreasonable data results in a loss of performance in the validation dataset relative to the calibration performance. Mistrust of hydrometric data limits the advancement of hydrologic science because an improved process understanding requires data that are trusted to be close to the truth.
It is a very good thing for the science and practice of hydrology that there are so many practitioners interested in learning how to develop better rating curves that are reliable, credible and defensible.
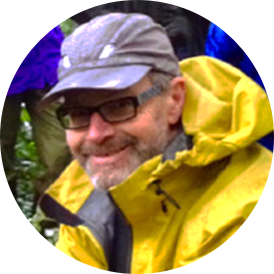